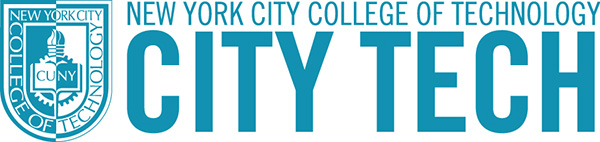
Publications and Research
Document Type
Article
Publication Date
Spring 4-11-2016
Abstract
Ensemble filtering techniques filter noisy instances by combining the predictions of multiple base models, each of which is learned using a traditional algorithm. However, in the last decade, due to the massive increase in the amount of online streaming data, ensemble filtering methods, which largely operate in batch mode and requires multiple passes over the data, cause time and storage complexities. In this paper, we present an ensemble bootstrap model filtering technique with multiple inductive learning algorithms on several small Poisson bootstrapped samples of online data to filter noisy instances. We analyze three prior filtering techniques using Bayesian computational analysis to understand the underlying distribution of the model space. We implement our and other prior filtering approaches and show that our approach is more accurate than other prior filtering methods.
Comments
This work was originally published in Information Technology: New Generations