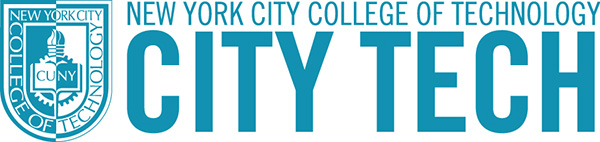
Publications and Research
Document Type
Article
Publication Date
Spring 4-8-2016
Abstract
In the last decade Data mining (DM) has been applied in the field of education, and is an emerging interdisciplinary research field also known as Educational Data Mining (EDM). One of the goals of EDM is to better understand how to predict student academic performance given personal, socio-economic, psychological and other environmental attributes. Another goal is to identify factors and rules that influence educational academic outcomes. In this paper, we use multiple classifiers (Decision Trees-J48, Naïve Bayes and Random Forest) to improve the quality of student data by eliminating noisy instances, and hence improving predictive accuracy. We also identify association rules that influence student outcomes using a combination of rule based techniques (Apriori, Filtered Associator and Tertius). We empirically compare our technique with single model based techniques and show that using ensemble models not only gives better predictive accuracies on student performance, but also provides better rules for understanding the factors that influence better student outcomes.
Comments
This work was originally presented at ASEE Mid-Atlantic Section Spring 2016 Conference, held at George Washington University, Washington D.C, April 8-9, 2016.