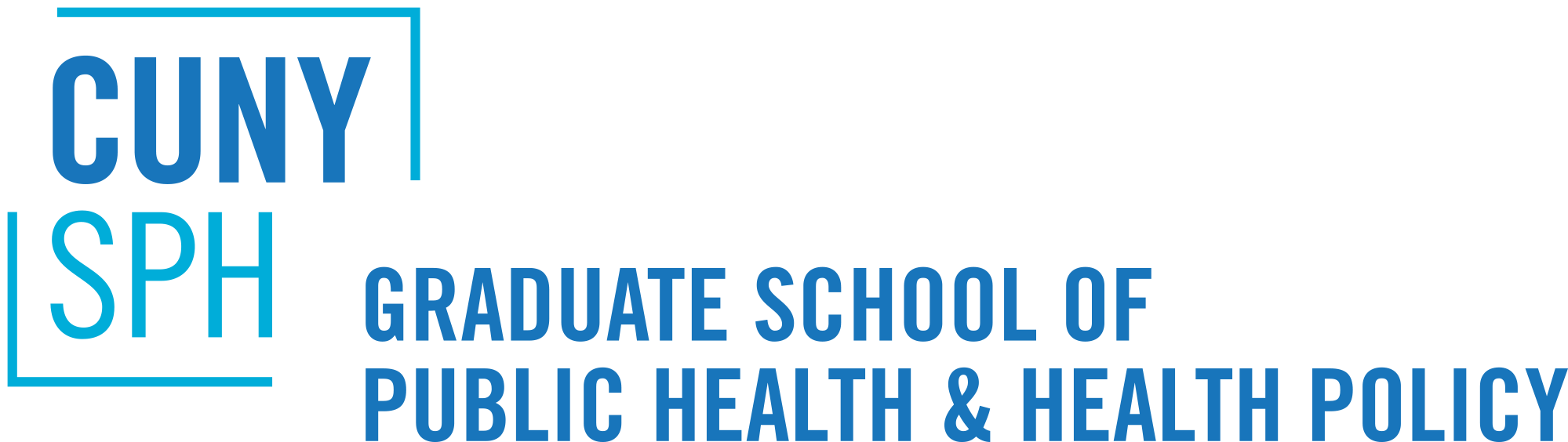
Dissertations and Theses
Date of Degree
6-1-2020
Document Type
Dissertation
Degree Name
Doctor of Philosophy (Ph.D.)
Department
Epidemiology and Biostatistics
Advisor(s)
Levi Waldron
Committee Members
Heidi Jones
Katarzyna Wyka
Jennifer Lighter
Subject Categories
Clinical Epidemiology | Health and Medical Administration | Health Information Technology | Patient Safety | Public Health
Keywords
healthcare, AI, prediction modeling
Abstract
Background
Hospital readmissions within 30 days after discharge have drawn national policy attention as they are a reflection of suboptimal patient care. Readmissions are costly, accounting for more than $17 billion in potentially avoidable Medicare expenditures - nearly 78% of readmissions may be avoidable. Rich electronic data from medical records, growing computing capacities, and open source machine learning algorithms offer new opportunities to predict patients at high risk for readmission and prevent readmission through focused interventions. Prediction models might also serve to provide a more nuanced context of patient characteristics that lead to variations in readmission rates. Furthermore, transitional care between hospitals and skilled nursing facilities is a critical component of patient readmission prevention management. Successful transitional care must include the development of a comprehensive care plan and the availability of experienced health practitioners who are provided relevant medical information on patients’ readmission risk.
Methods
Predictive models were developed using statistical and machine learning algorithms to identify patients at risk for readmission as well as readmissions associated with pneumonia, sepsis and urinary tract infections after discharge to skilled nursing facilities. Over 3,000 features associated with patients discharged to skilled nursing facilities were extracted from NYU Langone Heath’s electronic health record system, and analyzed using logistic regression, gradient boosting trees, support vector machine, and neural network algorithms. A time split-sample approach was used to partition the data into training, validation, and test sets according to year: 2012-2017 data for training (n = 9,725), 2018 data for validation (n=3,878) and 2019 data for test data (n = 4,342). The most accurate model was selected based on discrimination and calibration performance. The selected model for overall readmission risk was compared to previously published index score models using discrimination and calibration performance. A variable importance algorithm was used to determine the important features of the selected models for overall readmission and readmissions associated with infections. Lastly, using the risk estimates from the models with the four readmission outcomes, a notification and reporting system for key stakeholders was created, including a standardized readmission ratio comparing the observed to the expected number of readmissions by discharging provider and skilled nursing facility.
Results
A gradient boosting model was selected as the best model to predict overall readmission risk using only real-time data. Discrimination performance was better or similar to previously published index score models that rely on coded data, and calibration was superior. Gradient boosting models were also used to classify readmission risk associated with sepsis, pneumonia, and urinary tract infections. Risk estimates from the models were successfully used to calculate a Readmission Risk Ratio metric. This metric was incorporated into an email to notify key stakeholders and develop risk-adjusted reports.
Conclusions
Hospitals can leverage the rich data found in electronic health records to generate readmission prediction models optimized for their patient population. This study builds several predictions models, develops an artificial intelligence notification tool, and explores potential interventions as part of a broader program. It does not however asses the effectiveness of the tool nor the interventions’ effect on readmission rates. Validated models can be deployed to target resources for patients at high risk for readmission with proven interventional programs and facilitate collaboration among transitional care teams.
Recommended Citation
Stachel, Anna, "Development of a hospital readmission reduction program for patients discharged to skilled nursing facilities: An application of artificial intelligence and machine learning techniques" (2020). CUNY Academic Works.
https://academicworks.cuny.edu/sph_etds/59
Included in
Clinical Epidemiology Commons, Health and Medical Administration Commons, Health Information Technology Commons, Patient Safety Commons