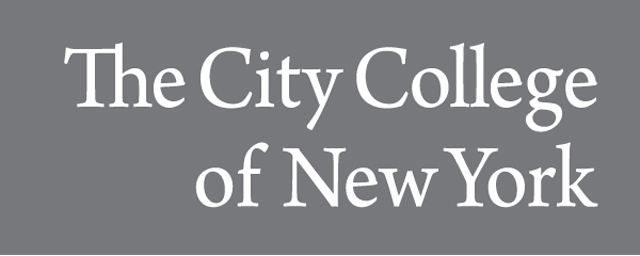
Dissertations and Theses
Date of Award
2022
Document Type
Dissertation
Department
Engineering
First Advisor
Yingli Tian
Keywords
Computer vision, Deep learning, Medical image analysis, Artificial intelligence, Computer-aided diagnosis
Abstract
Medical imaging has been applied widely in many clinical diagnoses to detect and differentiate abnormalities by revealing the internal structure of the human body at normal anatomical and physiological levels. Manual analyzing medical images demands attention and is time-consuming, requiring well-trained expertise. The speed, fatigue, and experience may limit the diagnostic performance, leading to delays and even false diagnoses that significantly impact patient treatment. Therefore, accurate systematic systems based on medical image analysis are crucial for timely clinical diagnosis.
This dissertation focuses on advancing automatic computer-aided diagnosis systems to detect cancer, assisting radiologists with early intervention to improve survival rates. Many researchers have employed Artificial intelligence (AI) in automatic diagnosis systems for cancer detection and assessment. However, existing work is still limited to safer medical imaging modalities, accurate automated cancer diagnosis, efficient image annotation acquisition, and robustness in different domains.
This dissertation aims to address the remaining concerns within the following four aspects: (1) Contrast-enhanced medical images for computer-aided diagnosis. It can effectively highlight the internal structure of blood vessels and organs, help abnormal detection, and is a better image representation. (2) Effective medical image annotation acquisition. It significantly saves the expensive annotation costs, accelerates the training process, and prevents AI model overfitting with limited training images. (3) Automatic cancer diagnosis system with high sensitivity and specificity. It is required to accurately detect, segment, and classify abnormality on medical images and can be adapted to multiple manufacturers of medical images. (4) Identifying out-of-distribution data by uncertainty estimation. It prevents the AI-driven diagnostic model from generating over-confident predictions due to image distribution shifts. This dissertation reviews the previous research for these tasks and remaining issues, as well as proposes AI-driven solutions to tackle these issues and presents the results.
Recommended Citation
Liu, Jingya, "AI-driven Automated Medical Imaging Analysis" (2022). CUNY Academic Works.
https://academicworks.cuny.edu/cc_etds_theses/1065