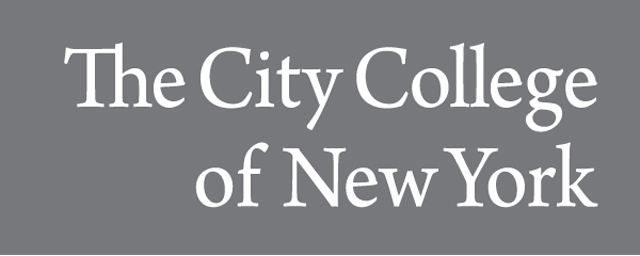
Publications and Research
Document Type
Article
Publication Date
4-5-2018
Abstract
Aim: Currently, identifying multiple sclerosis (MS) by human experts may come across the problem of “normal-appearing white matter”, which causes a low sensitivity. Methods: In this study, we presented a computer vision based approached to identify MS in an automatic way. This proposed method first extracted the fractional Fourier entropy map from a specified brain image. Afterwards, it sent the features to a multilayer perceptron trained by a proposed improved parameter-free Jaya algorithm. We used cost-sensitivity learning to handle the imbalanced data problem. Results: The 10 × 10-fold cross validation showed our method yielded a sensitivity of 97.40 ± 0.60%, a specificity of 97.39 ± 0.65%, and an accuracy of 97.39 ± 0.59%. Conclusions: We validated by experiments that the proposed improved Jaya performs better than plain Jaya algorithm and other latest bioinspired algorithms in terms of classification performance and training speed. In addition, our method is superior to four state-of-the-art MS identification approaches.
Comments
This work was originally published in entropy, available at https://doi.org/10.3390/e20040254.
This article is an open access article distributed under the terms and conditions of the Creative Commons Attribution (CC BY) license (http://creativecommons.org/licenses/by/4.0/).