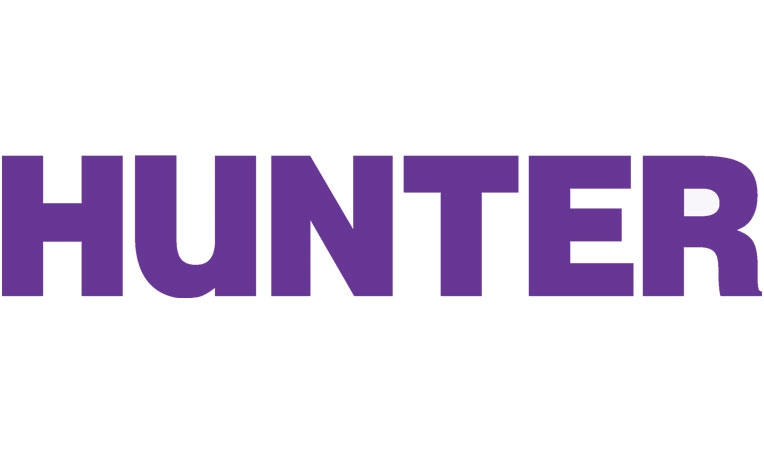
Publications and Research
Document Type
Article
Publication Date
8-15-2022
Abstract
Coronavirus disease 2019 (COVID-19) started in Wuhan, China, in late 2019, and after being utterly contagious in Asian countries, it rapidly spread to other countries. This disease caused governments worldwide to declare a public health crisis with severe measures taken to reduce the speed of the spread of the disease. This pandemic affected the lives of millions of people. Many citizens that lost their loved ones and jobs experienced a wide range of emotions, such as disbelief, shock, concerns about health, fear about food supplies, anxiety, and panic. All of the aforementioned phenomena led to the spread of racism and hate against Asians in western countries, especially in the United States. An analysis of social preliminary police data by the Center for the Study of Hate & Extremism at California State University shows that Anti-Asian hate crime in 16 of America’s largest cities increased by 149% in 2020. In this study, we first chose a baseline of Americans’ hate crimes against Asians on Twitter. Then we present an approach to balance the biased dataset and consequently improve the performance of tweet classification. We also have downloaded 10 million tweets through the Twitter API V-2. In this study, we have used a small portion of that, and we will use the entire dataset in the future study. In this article, three thousand tweets from our collected corpus are annotated by four annotators, including three Asian and one Asian- American. Using this data, we built predictive models of hate speech using various machine learning and deep learning methods. Our machine learning methods include Random Forest, K-nearest neighbors (KNN), Support Vector Machine (SVM), Extreme Gradient Boosting (XGBoost), Logistic Regression, Decision Tree, and Naive Bayes. Our Deep Learning models include Basic Long-Term Short-Term Memory (LSTM), Bidirectional LSTM, Bidirectional LSTM with Drop out, Convolution, and Bidirectional Encoder Representations from Transformers (BERT). We also adjusted our dataset by filtering tweets that were ambiguous to the annotators based on low Fleiss Kappa agreement between annotators. Our final result showed that Logistic Regression achieved the best statistical machine learning performance with an F1 score of 0.72, while BERT achieved the best performance of the deep learning models, with an F1-Score of 0.85.
Comments
This article was originally published in Frontiers in Artificial Intelligence and can be accessed at https://doi.org/10.3389/frai.2022.932381.
This is an open-access article distributed under the terms of the Creative Commons Attribution License (CC BY). The use, distribution or reproduction in other forums is permitted, provided the original author(s) and the copyright owner(s) are credited and that the original publication in this journal is cited, in accordance with accepted academic practice. No use, distribution or reproduction is permitted which does not comply with these terms.