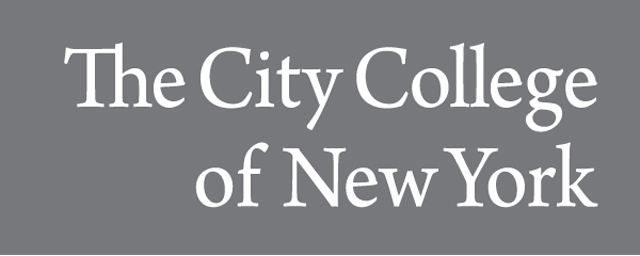
Dissertations and Theses
Date of Award
2023
Document Type
Thesis
Department
Computer Science
First Advisor
Allison Bishop
Second Advisor
Akira Kawaguchi
Keywords
Differential Privacy, Modeling, Stock Data, Distributions, Trading
Abstract
Information leakage is a major concern for traders who want to execute large orders without affecting the market price. In this paper, we explore the sources and effects of information leakage in historical stock market data using various methods and metrics. We first define information leakage as a pattern caused by a trader that would otherwise not occur without the traderโs activity. Using historical data, the direct impact of a potential large trade cannot be measured, but we consider a minimal impact large trade to be one that minimizes changes to the established trading data. We then analyze how information leakage varies across different stocks, and the degree of influence a potential large trade may have on a stock. We also create a method for setting a policy driven bound using ๐^๐ in the spirit of differential privacy and investigate how an ๐-bound would affect trade-offs in information leakage and speed. Finally, we propose some strategies to reduce information leakage and improve trading efficiency of large trades.
Recommended Citation
Hua, Edison, "Exploring Information Leakage in Historical Stock Market Data" (2023). CUNY Academic Works.
https://academicworks.cuny.edu/cc_etds_theses/1147
Included in
Data Science Commons, Finance Commons, Risk Analysis Commons, Statistical Models Commons