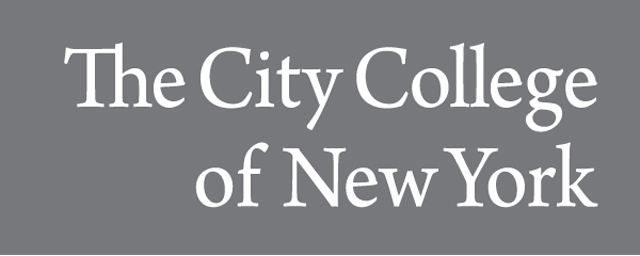
Dissertations and Theses
Date of Award
2022
Document Type
Dissertation
Department
Civil Engineering
First Advisor
Reza Khanbilvardi
Second Advisor
Tarendra Lakhankar
Third Advisor
Mimi Hughes
Keywords
snow, streamflow, snow water equivalent, National Water Model, WRF-Hydro, Noah-MP
Abstract
Snow has great influence on land-atmosphere interactions and snowmelt from the mountains is a vital water source for downstream communities dependent on snow fed lakes, rivers and streams. This study explored the snow and streamflow prediction capabilities of process-based numerical prediction and data-driven machine learning models.
The overall goal of this study was to understand the deficiencies in the NOAA’s National Water Model (NWM) to represent snow, subsequently streamflow, and recognize the areas where it could be improved for future model developments. The goal was also to evaluate if the recent advancements in machine learning techniques is useful for predicting snow in mountainous terrain where numerical prediction models such as the NWM have been known to face difficulties.
The first chapter of this document will give an overview of the history of process-based hydrological models from their inception to a highly complex numerical expression of fully distributed models. It also describes the most recent evolution of more data-driven machine learning (ML) approaches such as artificial recurrent neural networks that are able to learn the non-linear input-output hydrological relationships in a catchment without explicit physical representation of the processes. This will help in understanding the vices and virtues of each method.
The second chapter of this document will evaluate snow representation in the NWM through a single-column experiment and subsequently streamflow across the Aroostook River Basin in northeastern Maine, United States. It will analyze the uncertainties in the meteorological forcing data and model biases that lead to biases in the snow representation in the model simulations. The simulated streamflow estimates will be compared against the observed values from the United States Geological Survey and highlight the factors that lead to the discrepancies. The insights from the single-column and the basin study will help in the future development of the NWM or other process-based physical numerical prediction hydrological models.
The third chapter of this document will evaluate the long short-term memory network machine learning technique to predict the daily snow water equivalent SWE in the Sierra Nevada basins in the western United States. This chapter takes advantage of a newly developed unique SWE reanalysis dataset, and compares SWE predicted with the ML technique to the SWE estimates from the physically based National Water Model. Snow in the Sierra Nevada range in the Western United States is critical for providing water to most parts of the south western United States, the regions that are facing severe drought since the past decade. The results of this study will be valuable towards the ongoing research on ways to more accurately estimate and predict snow stored in the mountains as SWE every season.
Recommended Citation
Sthapit, Engela, "Evaluation of Snow and Streamflow in the National Water Model with Analysis using Machine Learning" (2022). CUNY Academic Works.
https://academicworks.cuny.edu/cc_etds_theses/1037