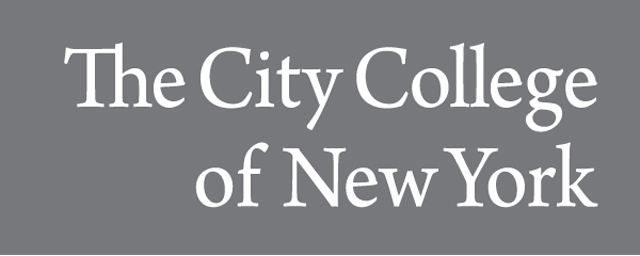
Dissertations and Theses
Date of Award
2024
Document Type
Dissertation
Department
Mechanical Engineering
First Advisor
Hao Su
Second Advisor
Honghui Yu
Keywords
Pediatric exoskeleton, actuator optimization, collocated control, cerebral palsy
Abstract
Lightweight and smart exoskeletons offer the potential to improve mobility in children. State-of-the-art pediatric exoskeletons are typically clinic-based since they are either tethered or portable but cumbersome, and their design is often not optimized across a range of environments and users. Child growth progressively alters key exoskeleton design requirements, yet no prior work investigated actuator optimization to accommodate child growth. Moreover, exoskeletons typically use the torque sensor or spring mechanics as exteroceptive feedback, but these torque sensing methods not only lead to extra cost and mass but also cause a small range of stable controller gains.
To facilitate pediatric exoskeleton in community settings, firstly, we proposed an actuator optimization framework that identified the optimal design parameters for both motor and transmission while minimizing the actuator mass and satisfying the output torque, speed, bandwidth, and resistance torque requirements. Guided by the optimization results, this thesis proposes a lightweight (1.78 kg unilateral mass) and user-specific, fully portable pediatric knee exoskeleton for children. Secondly, we created a unified architecture for torque-controlled pediatric exoskeletons that can compare either Series Elastic Actuator (SEA) or Quasi-Direct Drive (QDD) actuator schemes with different sensing methods, providing a unified method to analyze their stability systematically. Following this architecture, we developed a collocated controller integrated with a torque estimator for QDD-based exoskeletons, enhancing their stability over non-collocated SEA and QDD-based exoskeleton architectures. Thirdly, this work developed a new algorithm, namely, Long Short Term Memory (LSTM)-based gait phase detection for children with cerebral palsy (non-cyclic gaits, while the state-of-art only detect cyclic gaits).
The experimental results on four adults and one child demonstrated that our exoskeleton has high compliance (0.30 Nm root mean square resistive torque) and high bandwidth (40.3 Hz). Experimental results comparing our collocated control architecture to state-of-the-art non-collocated architecture showed an increase in the stability region (our 800 Nm/rad vs. state-of-the-art 300 Nm/rad in 2.0 Hz) and torque estimation accuracy compared to a current-based torque estimator (error reduced from 10%-20% to 3%-5%). These results enable the implementation of higher joint stiffness in exoskeletons for tasks such as running (knee joint requirement: 332–511 Nm/rad at 2.0 m/s speed). The models accurately estimated the gait phase on irregular walking patterns (accuracy 94.60\%) without explicit training in children (typically developing and with cerebral palsy). The controller results demonstrated an elevated ability to adapt to the irregular gait patterns of the child with cerebral palsy. In summary, this work presented a holistic solution that includes both hardware innovation (actuator optimization framework and non-collocated/collocated architecture) and software innovation (collocated control and deep learning-based control) toward the paradigm shift of pediatric exoskeletons from clinic to community setting.
Recommended Citation
Zhang, Sainan, "Actuator Optimization and Control of Pediatric Knee Exoskeleton for Community-based Mobility Assistance: More Lightweight, More Affordable, and More Stable" (2024). CUNY Academic Works.
https://academicworks.cuny.edu/cc_etds_theses/1130