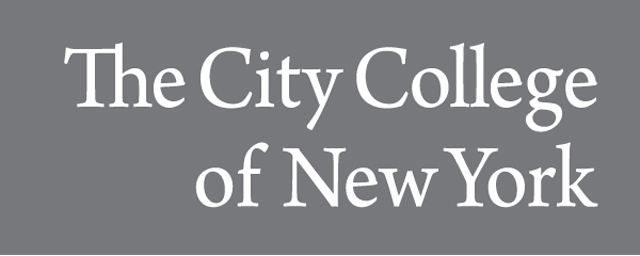
Dissertations and Theses
Date of Award
2023
Document Type
Dissertation
Department
Engineering
First Advisor
Jizhong Xiao
Abstract
Ground Penetrating Radar (GPR) serves as a significant nondestructive evaluation (NDE) technique to inspect and survey underground objects (e.g., rebars, utility pipes) in complex urban environments. In addition to GPR, visual-based surface defect detection plays a crucial role in assessing and maintaining civil infrastructure. To facilitate deployment on a robotic platform and enhance inspection capabilities, the integration of GPR and visual-based sensors, such as RGB-D cameras, is crucial to obtain accurate subsurface and surface information. This thesis presents a novel robotic-based inspection system for the efficient collection of NDE data and accurate 3D reconstruction of both subsurface and surface infrastructure elements.
The proposed system consists of four main components: 1) a robotic data collection module that enables free-motion GPR scanning and real-time tagging of metric positioning with GPR measurements; 2) an improved GPR imaging method that cleans noisy data in GPR B-scan images, enhancing data quality for more accurate interpretation; 3) a learning-based method for subsurface object 3D reconstruction from B-scan data without preprocessing, generating a volumetric occupancy map of subsurface objects; and 4) the development of visual inspection software for civil engineers to visualize, label, and measure surface defects from collected RGB and Depth images.
This thesis reviews various solutions proposed by researchers to address the challenges in robotic-based inspection and data analytics, demonstrates the proposed solutions and contributions, presents preliminary results, and outlines future work. The developed frameworks and methodologies have the potential to significantly impact the field of NDE analysis and facilitate more accurate and efficient NDE inspection across various application domains.
Recommended Citation
Feng, Jinglun, "Robotic Inspection and Data Analytics to Localize and Visualize the Structural Defects of Civil Infrastructure" (2023). CUNY Academic Works.
https://academicworks.cuny.edu/cc_etds_theses/1138