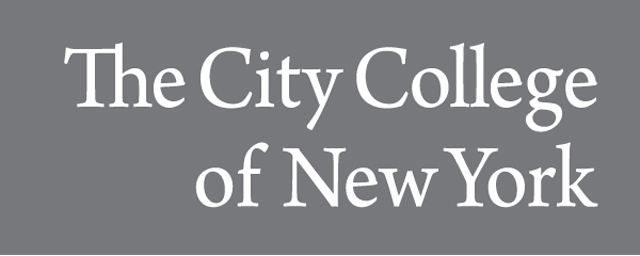
Dissertations and Theses
Date of Award
2023
Document Type
Thesis
Department
Computer Science
First Advisor
Hao Tang
Second Advisor
Zhigang Zhu
Keywords
Machine Learning, Sensor Design, Multi-modal Data, Audio Data Processing, Assistive Navigation, Blind or Low Vision
Abstract
Navigating safely and independently presents considerable challenges for people who are blind or have low vision (BLV), as it requires a comprehensive understanding of their neighborhood environment. Our user study reveals that materials and objects on sidewalks play a crucial role in navigation tasks. Unfortunately, current methods for assessing sidewalk materials are suboptimal, often relying on labor-intensive and expensive manual assessments that fail to capture the full range of sidewalk features critical to individuals with BLV.
In response to this problem, this master’s thesis investigates deep learning approaches specifically designed for the classification of multi-modal sidewalk materials. The proposed framework aims to empower individuals with BLV to automatically gather information about sidewalk materials while navigating their surroundings. This innovative solution comprises two primary components. (1) First, the study focuses on designing a lightweight data collection methodology that involves attaching an inertial measurement unit (IMU) and a microphone to the white cane. This sensor design enables the measurement of the haptic and audio feedback, represented by acceleration data and audio data, respectively, as the white cane interacts with the sidewalk surface. The collected acceleration and acoustic signal data effectively capture the unique characteristics of different sidewalk materials. Utilizing this novel data collection method, we have successfully generated a multi-modal sidewalk material (MSM) dataset, encompassing a wide range of sidewalk material categories. (2) the research develops a deep learning-based classifier to identify different sidewalk materials using this multi-modal data. We investigate two model architectures: the ResNet-Encoder model and the Transformer-Encoder model to understand their efficacy in sidewalk material classification. Experimental results indicate that the ResNet-Encoder model provides superior performance, achieving an optimal accuracy of 83\% when trained with 4-second-long data clips.
In summary, our research has significant implications for the development of AI-based assistive navigation solutions for individuals with BLV. It contributes to both the methodology for sidewalk material data collection and the algorithm of deep learning for sidewalk material classification. By employing the proposed multi-modal deep learning approach, BLV people can effortlessly acquire information about sidewalk materials. Furthermore, this data can be utilized to generate an urban accessibility geospatial map, thereby facilitating independent travel for individuals with BLV.
Recommended Citation
Liu, Jiawei, "Classifying Sidewalk Materials Using Multi-Modal Data" (2023). CUNY Academic Works.
https://academicworks.cuny.edu/cc_etds_theses/1142