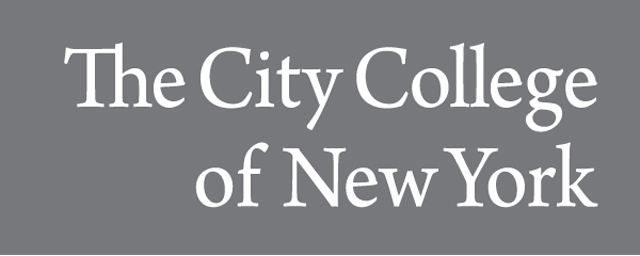
Dissertations and Theses
Date of Award
2023
Document Type
Thesis
Department
Computer Science
First Advisor
Karin Block
Second Advisor
Michael Grossberg
Keywords
Basalts, Chemical Features, Basalt Chemistry, Random Forest, Machine Learning, Outlier Detection, Ternary Plots, RFE, Model Interpretation, Outlier Ensemble Model
Abstract
Scientists use basalt chemistry to discriminate among different tectonic settings. There are well-known chemical elements used to classify tectonic settings. An exploration of new features is done using Logistic Regression and Random Forest to discover any new elements of interest. The models were used with other tools, such as recursive feature elimination and permutations, to increase reliability. Among the scarcely explored chemical elements are Terbium (Tb), Holmium (Ho), Samarium (Sm), and Erbium (Er). The data used for the exploration contained many outliers. Therefore, an ensemble model was created to explore the location and composition of such outliers. The ensemble was tested with synthetic data to measure performance. The synthetic data with the same distribution as the underlying data showed an accuracy of 73%, while other distributions of synthetic data reached up to 98% accuracy.
Recommended Citation
Vivar, Jenifer, "Analysis of Chemical Elements in Basalts using Mislabeled Data, a Machine Learning Approach" (2023). CUNY Academic Works.
https://academicworks.cuny.edu/cc_etds_theses/1146