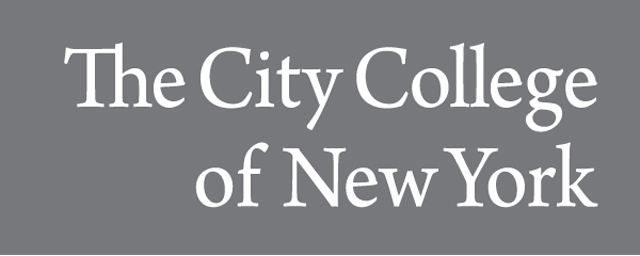
Dissertations and Theses
Date of Award
2019
Document Type
Dissertation
Department
Engineering
First Advisor
Barry Gross
Keywords
Crop Growth Models, Remote Sensing, LAI
Abstract
Agricultural systems are difficult to model because crop growth is driven by the strongly nonlinear interaction of Genotype x Environment x Management (G x E x M) factors. Due to the nonlinearity in the interaction of these factors, the amount of data necessary to develop and utilize models to accurately predict the performance of agricultural systems at an operational scale is large. Satellite remote sensing provides the potential to vastly increase the amount of data available for modelling agricultural systems as a result of its high revisit time and spatial coverage. Unfortunately, there have been significant difficulties in deploying remote sensing for many agricultural modelling applications because of the uncertainty involved in the retrievals. In this dissertation, we show that collecting farmer-provided agro-managment information has the potential to reduce the uncertainty in the retrieval products obtained from remote sensing observations. Specifically, both field-scale and regional-scale analysis are used to show that secondary factor variability is a very significant cause of uncertainty in both crop growth modelling and agricultural remote sensing that needs to be addressed through increased data collection. In order to address this need for increased data availability, a method is developed that allows geolocated crop growth model simulations to be used to train satellite-based crop state variable retrievals, which is then validated at regional scale. The method developed provides a general robust methodology to create a large-scale platform that would allow farmers to share data with government agencies and universities to improve crop state variable retrievals and crop growth modelling and provide farmers, government, industry, and researchers with insights and predictive capability into crop growth at both field and regional scales.
Recommended Citation
Levitan, Nathaniel, "Improving Retrievals of Crop Vegetation Parameters from Remote Sensing Data" (2019). CUNY Academic Works.
https://academicworks.cuny.edu/cc_etds_theses/885
Included in
Bioresource and Agricultural Engineering Commons, Electrical and Computer Engineering Commons, Environmental Engineering Commons