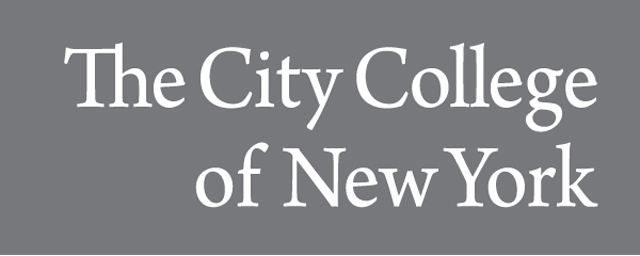
Publications and Research
Document Type
Article
Publication Date
September 2014
Abstract
Severe pediatric sepsis continues to be associated with high mortality rates in children. Thus, an important area of biomedical research is to identify biomarkers that can classify sepsis severity and outcomes. The complex and heterogeneous nature of sepsis makes the prospect of the classification of sepsis severity using a single biomarker less likely. Instead, we employ machine learning techniques to validate the use of a multiple biomarkers scoring system to determine the severity of sepsis in critically ill children. The study was based on clinical data and plasma samples provided by a tertiary care center's Pediatric Intensive Care Unit (PICU) from a group of 45 patients with varying sepsis severity at the time of admission. Canonical Correlation Analysis with the Forward Selection and Random Forests methods identified a particular set of biomarkers that included Angiopoietin-1 (Ang-1), Angiopoietin-2 (Ang-2), and Bicarbonate (HCO) as having the strongest correlations with sepsis severity. The robustness and effectiveness of these biomarkers for classifying sepsis severity were validated by constructing a linear Support Vector Machine diagnostic classifier. We also show that the concentrations of Ang-1, Ang-2, and HCO enable predictions of the time dependence of sepsis severity in children.
Comments
This work was originally published in PLoS ONE, available at doi:10.1371/journal.pone.0108461.