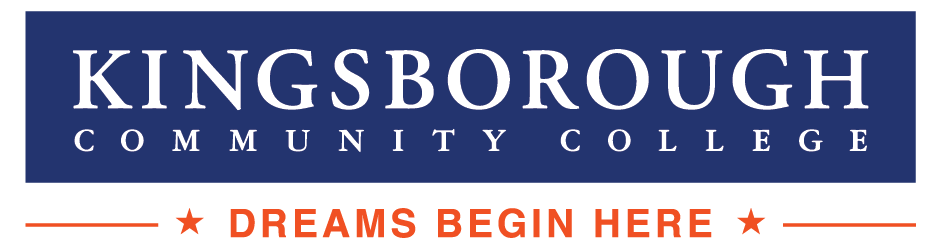
Publications and Research
Document Type
Article
Publication Date
2013
Abstract
Ordinary least-squares regression suffers from a fundamental lack of symmetry: the regression line of y given x and the regression line of x given y are not inverses of each other. Alternative symmetric regression methods have been developed to address this concern, notably: orthogonal regression and geometric mean regression. This paper presents in detail a variety of least squares regression methods which may not have been known or fully explicated. The derivation of each method is made efficient through the use of Ehrenberg's formula for the ordinary least-squares error and through the extraction of a weight function g(b) which characterizes the regression. For every case of generalized least-squares, the error between the line and the data is shown to be a product of the weight function g(b) and Ehrenberg's error formula.
Included in
Mathematics Commons, Numerical Analysis and Computation Commons, Statistics and Probability Commons
Comments
This work was originally published in Proceedings of the 1st International Conference on Computational Science and Engineering (CSE'13.