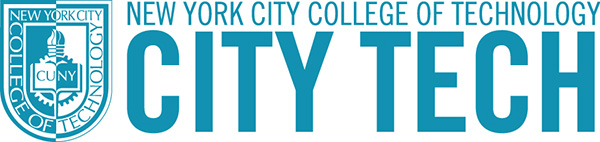
Publications and Research
Document Type
Article
Publication Date
5-21-2021
Abstract
Chromosomal instability (CIN) is a hallmark of human cancer yet not readily testable for patients with cancer in routine clinical setting. In this study, we sought to explore whether CIN status can be predicted using ubiquitously available hematoxylin and eosin histology through a deep learning-based model. When applied to a cohort of 1,010 patients with breast cancer (Training set: n = 858, Test set: n = 152) from The Cancer Genome Atlas where 485 patients have high CIN status, our model accurately classified CIN status, achieving an area under the curve of 0.822 with 81.2% sensitivity and 68.7% specificity in the test set. Patch-level predictions of CIN status suggested intra-tumor heterogeneity within slides. Moreover, presence of patches with high predicted CIN score within an entire slide was more predictive of clinical outcome than the average CIN score of the slide, thus underscoring the clinical importance of intra-tumor heterogeneity.
Included in
Analytical, Diagnostic and Therapeutic Techniques and Equipment Commons, Artificial Intelligence and Robotics Commons, Biomedical Informatics Commons, Oncology Commons
Comments
This article was originally published in iScience, available at https://doi.org/10.1016/j.isci.2021.102394
This work is distributed under a Creative Commons Attribution 4.0 International License (CC BY 4.0).