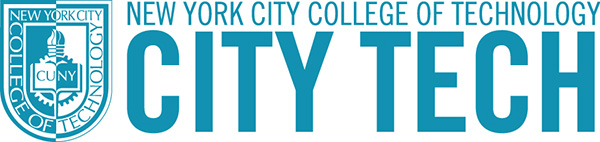
Publications and Research
Document Type
Poster
Publication Date
5-5-2021
Abstract
Indoor localization is difficult because of issues regarding the physics of signal propagation and multipath interference. Satellite-based technologies such as Global Positioning Systems (GPS) are unreliable indoors due to the signal attenuation during propagation and the multi-path interference. A data-driven process using the massive amounts of data available in smart devices may be the solution to this problem as they don’t rely on sophisticated indoor propagation models like traditional methods. The emergence of methods in machine learning society can be used to fulfill this task. The objective is to estimate the location of a person in a room using Received Signal Strength Indicator (RSSI). A public dataset containing the location of 13 ibeacons and their RSSI at various locations was used to accomplish this; the dataset consisted of one labeled set and one unlabeled set. The labeled set is used to train a machine learning model and the unlabeled set is used to evaluate the performance of the trained model; training the program to read the dataset should eventually lead to it being able to tell where a person is in a room given a unique dataset. The first phase of this project is to explore the feasibility of using data-driven approaches by visualizing the data then finding the correlations between the locations and the RSSI values. This has many applications such as alibi confirmations, asset tracking and contact tracing illnesses like Covid-19.
Comments
This poster, second place winner for STEM individual projects, was presented at the 34th Semi-Annual Dr. Janet Liou-Mark Honors & Undergraduate Research Poster Presentation, May 5, 2021. Mentor: Prof. Li Geng (Electrical and Telecommunications Engineering Technology).