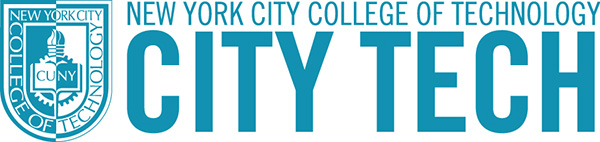
Publications and Research
Document Type
Article
Publication Date
11-18-2021
Abstract
Increasing usage of Digital Manufacturing (DM) in safety-critical domains is increasing attention on the cybersecurity of the manufacturing process, as malicious third parties might aim to introduce defects in digital designs. In general, the DM process involves creating a digital object (as CAD files) before using a slicer program to convert the models into printing instructions (e.g. g-code) suitable for the target printer. As the g-code is an intermediate machine format, malicious edits may be difficult to detect, especially when the golden (original) models are not available to the manufacturer. In this work we aim to quantify this hypothesis through a red-team/blue-team case study, whereby the red-team aims to introduce subtle defects that would impact the properties (strengths) of the 3D printed parts, and the blue-team aims to detect these modifications in the absence of the golden models. The case study had two sets of models, the first with 180 designs (with 2 compromised using 2 methods) and the second with 4320 designs (with 60 compromised using 6 methods). Using statistical modelling and machine learning (ML), the blue-team was able to detect all the compromises in the first set of data, and 50 of the compromises in the second.
Comments
Beckwith, Caleb, Harsh Sankar Naicker, Svara Mehta, Viba R. Udupa, Nghia Tri Nim, Varun Gadre, Hammond Pearce, Gary Mac, and Nikhil Gupta. "Needle in a Haystack: Detecting Subtle Malicious Edits to Additive Manufacturing G-code Files." IEEE Embedded Systems Letters (2021). © 2021 IEEE. Personal use of this material is permitted. Permission from IEEE must be obtained for all other uses, in any current or future media, including reprinting/republishing this material for advertising or promotional purposes, creating new collective works, for resale or redistribution to servers or lists, or reuse of any copyrighted component of this work in other works.
Funding: 10.13039/100000001-National Science Foundation (Grant Number: IRES OISE-1952479 and SaTC DGE-1931724), SecureAmerica Institute