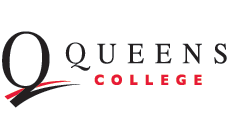
Publications and Research
Document Type
Article
Publication Date
2022
Abstract
The objective of this research is to investigate the feasibility of applying behavioral predictive analytics to optimize patient engagement in diabetes self-management, and to gain insights on the potential of infusing a chatbot with NLP technology for discovering health-related social needs. In the U.S., less than 25% of patients actively engage in self-health management even though self-health management has been reported to associate with improved health outcomes and reduced healthcare costs. The proposed behavioral predictive analytics relies on manifold clustering to identify subpopulations segmented by behavior readiness characteristics that exhibit non-linear properties. For each subpopulation, an individualized auto-regression model and a population-based model were developed to support self-management personalization in three areas: glucose self-monitoring, diet management, and exercise. The goal is to predict personalized activities that are most likely to achieve optimal engagement. In addition to actionable self-health management, this research also investigates the feasibility of detecting health-related social needs through unstructured conversational dialog. This paper reports the result of manifold clusters based on 148 subjects with type 2 diabetes and shows the preliminary result of personalization for 22 subjects under different scenarios, and the preliminary results on applying Latent Dirichlet Allocation to the conversational dialog of 10 subjects for discovering social needs in five areas: food security, health (insurance coverage), transportation, employment, and housing.
Included in
Artificial Intelligence and Robotics Commons, Endocrinology, Diabetes, and Metabolism Commons, Preventive Medicine Commons, Statistical Models Commons
Comments
This version of the article has been accepted for publication, after peer review (when applicable) and is subject to Springer Nature’s AM terms of use, but is not the Version of Record and does not reflect post-acceptance improvements, or any corrections. The Version of Record is available online at: http://dx.doi.org/10.1007/s42979-022-01092-2