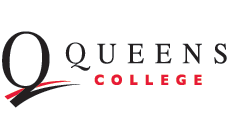
Publications and Research
Document Type
Article
Publication Date
2022
Abstract
We propose a new deep convolutional cephalometric landmark detection framework for orthodontic treatment. Our proposed method consists of two major steps: landmark detection using a deep neural network for object detection, and landmark repair to ensure one instance per landmark class. For landmark detection, we modify the loss function of the backbone network YOLOv3 to eliminate the constrains on the bounding box and incorporate attention mechanism to improve the detection accuracy. For landmark repair, a triangle mesh is generated from the average face to eliminate superfluous instances, followed by estimation of missing landmarks from the detected ones using Laplacian Mesh. Trained and evaluated on a public benchmark dataset from IEEE ISBI 2015 grand challenge, our proposed framework obtains comparable results compared to the state-of-the-art methods for cephalometric landmark detection, and demonstrates the efficacy of using a deep CNN model for accurate object detection of landmarks defined by only a single pixel location.
Comments
Originally published as: King, Cheng-Ho, Yin-Ling Wang, and Chia-Ling Tsai. "Automatic Cephalometric Landmark Detection on X-Ray Images Using Object Detection." IEEE International Symposium on Biomedical Imaging, 2022.
© 20XX IEEE. Personal use of this material is permitted. Permission from IEEE must be obtained for all other uses, in any current or future media, including reprinting/republishing this material for advertising or promotional purposes, creating new collective works, for resale or redistribution to servers or lists, or reuse of any copyrighted component of this work in other works.