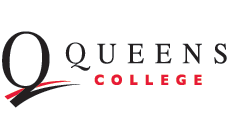
Publications and Research
Document Type
Article
Publication Date
7-2022
Abstract
In 2019, New York State Education Department announced 54.6% of all students in grades 3 to 8 not meeting the standard of reading proficiency. Motivated by the need for a more efficient intervention model, we propose a recommender system to leverage the technology in machine learning to recommend suitable reading materials for effective intervention. The recommendation is based on the student's prior reading comprehension assessments and also assessments of other students at the same grade level using collaborative filtering. No other prior academic or demographic information of students is available. Two main challenges are lack of explicit ratings of reading passages by students and the small data size. Both are addressed in this paper. BERT is applied to determine the textual evidence of a question, and linguistic properties are extracted to generate a continuous rating for a question answered by a student to reflect the skill level of the student. The difficulty level of a passage is determined by the associated multiple-choice questions. The system is trained with a collection of fourth grade New York English Language Arts assessments. The training dataset is augmented with synthetic data using SMOTE for better generalizability. Our system achieves 75.7% in accuracy and 59.23% in F1-score.
Comments
This version of the contribution has been accepted for publication, after peer review (when applicable) but is not the Version of Record and does not reflect post-acceptance improvements, or any corrections. Use of this Accepted Version is subject to the publisher’s Accepted Manuscript terms of use https://www.springernature.com/gp/open-research/policies/accepted-manuscript-terms