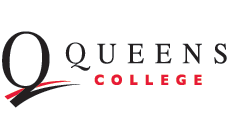
Publications and Research
Document Type
Article
Publication Date
2022
Abstract
Abstract—A model’s interpretability is essential to many practical applications such as clinical decision support systems. In this paper, a novel interpretable machine learning method is presented, which can model the relationship between input variables and responses in humanly understandable rules. The method is built by applying tropical geometry to fuzzy inference systems, wherein variable encoding functions and salient rules can be discovered by supervised learning. Experiments using synthetic datasets were conducted to demonstrate the performance and capacity of the proposed algorithm in classification and rule discovery. Furthermore, we present a pilot application in identifying heart failure patients that are eligible for advanced therapies as proof of principle. From our results on this particular application, the proposed network achieves the highest F1 score. The network is capable of learning rules that can be interpreted and used by clinical providers. In addition, existing fuzzy domain knowledge can be easily transferred into the network and facilitate model training. In our application, with the existing knowledge, the F1 score was improved by over 5%. The characteristics of the proposed network make it promising in applications requiring model reliability and justification.
Comments
This is the accepted manuscript of an article finally published as: Yao, Heming. Harm Derksen, Jessica R. Golbus, Justin Zhang, Keith D. Aaronson, Jonathan Gryak, and Kayvan Najarian. "A Novel Tropical Geometry-based Interpretable Machine Learning Method: Pilot Application to Delivery of Advanced Heart Failure Therapies." IEEE Journal of Biomedical and Health Informatics. doi: 10.1109/JBHI.2022.3211765
This work is licensed under a Creative Commons Attribution-NonCommercial-NoDerivatives 4.0 International License.