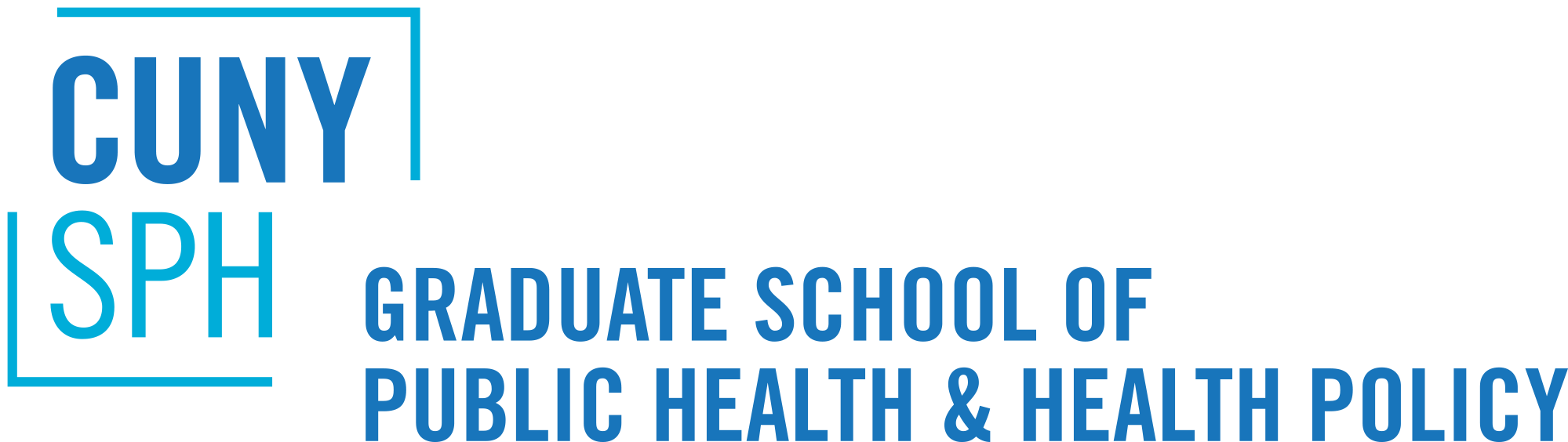
Dissertations and Theses
Date of Degree
6-1-2024
Document Type
Dissertation
Degree Name
Doctor of Philosophy (Ph.D.)
Department
Epidemiology and Biostatistics
Advisor(s)
Denis Nash
Committee Members
Till Barnighausen
McKaylee Robertson
Anne Schuster
Chloe Teasdale
Subject Categories
Epidemiology | Public Health
Keywords
COVID-19, population-based surveys, bias, prevalence, surveillance
Abstract
BACKGROUND: Population-based surveys designed to randomly sample the population can be a critical and complementary tool to traditional surveillance approaches. Probability-based surveys enable the measurement of SARS-CoV-2 testing and outcomes that avoid issues around self-selection into testing, making them an effective approach to address ascertainment bias in passive surveillance. For surveys to be a useful and reliable surveillance tool for understanding the burden and distribution of SARS-CoV-2 infection, they need to capture prevalence estimates that are both valid and reliable to be optimally informative for public health measures. The dissertation was undertaken to address three main gaps that have implications on the validity and reliability of population-based burden of infection .
METHODS: The dissertation aimed to assess population characteristics associated with testing with a provider and exclusive home-based testing (Aim 1) and describe factors associated with testing uptake and testing preferences. A population-based representative survey that randomly sampled New York City adults was used to characterize SARS-CoV-2 testing uptake by testing types across socio-economic characteristics of NYC neighborhoods. Second, the dissertation applied a Hybrid Prevalence Estimation (HPE) methodology (Aim 2) by triangulating probability-based surveys to model testing propensity to adjust biased provider-based testing and cases reported through passive surveillance. Third, the dissertation applied Heckman-type selection models (Aim 3) using a randomly assigned micro-incentive to investigate the presence and extent of non-ignorable non-response bias on SARS-CoV-2 prevalence estimates from a routinely administered point SARS-CoV-2 prevalence survey.
RESULTS: For the first aim, we found that Black non-Hispanic (41.4%, 95% CI 29.5, 53.4) and Asian American and Pacific Islander adults (43.1%, 95% CI 24.6, 61.6) were least likely to test overall (provider or home-based testing) compared to non-Hispanic white adults (58.5%, 95% CI 51.3, 65.7) and Hispanic adults (61.9%, 95% CI 51.7, 72.0). Non-Hispanic Black adults were less likely than non-Hispanic white adults to test exclusively at home (PR 0.5, 95% CI 0.2, 0.9) as were non-U.S. born (PR 0.7, 95% CI 0.4, 0.9) compared to U.S. born adults. Preference for provider-based testing was higher among non-Hispanic Black adults (50.4%, 95% CI 37.6, 63.3), those with a high school degree (52.1%, 95% CI 40.9, 63.4), adults with a household income between $25,000 – $65,000 (41.1%, 95% CI 31.6, 50.6), uninsured (45.6%, 95% CI 32.8, 58.3), unemployed (40.1%, 95% CI 33.2, 47.1), and non-U.S. born adults (39.2%, 95% 29.5, 48.8).
In the second aim, overall average propensity to test with a provider among NYC adults was 43.0% (95% CI 40.2, 45.9) citywide, 42.5% (95% CI 39.8, 46.6) in the Bronx, 44.7% (95% CI 41.6, 47.8) in Brooklyn, 39.5% (95% CI 35.8, 43.2) in Manhattan, 43.8% in Queens (95% CI 40.3, 47.3), and 42.9% (95% CI 39.8, 47.8) in Staten Island. Survey-based neighborhood-level SARS-CoV-2 prevalence estimates were higher than surveillance-based test positivity in 26 of the 34 neighborhoods, with test positivity point estimates falling outside the confidence limits of survey-based prevalence in five of the 34 neighborhoods. Compared to surveillance-based test positivity, HPE-based SARS-CoV-2 prevalence estimates were higher than test positivity in 29 of the 34 neighborhoods. The median value of half-width of 95% CI was 12.7% for survey-based estimates compared to 7.1% for HPE-based estimates. The HPE methodology yielded neighborhood SARS-CoV-2 prevalence estimates that were more precise than survey-based estimates with an average standard error of 0.04 compared to 0.09 for survey-based estimates.
In the third aim, compared to the naïve (unadjusted) SARS-CoV-2 prevalence of 14.9% (95% CI: 13.8, 16.0) among complete cases and an imputation-based adjusted prevalence of 15.2% (95% CI 14.2, 16.1), Heckman-type selection model under the Copula distribution showed a marginally statistically significant correlation between the survey response and outcome models (Tau = 0.30, 95% CI 0.003, 0.94) with a lower adjusted prevalence estimate of 13.0% (95% CI 8.3, 19.1).
DISCUSSION: This dissertation demonstrated the application of approaches using population-based surveys that fill key knowledge gaps when measuring burden of infection for pandemic planning and response. Population-based surveys can provide a representative assessment of testing behaviors, integrate population-representative testing propensity metrics to improve precision of infection burden at local scale, while being amenable to statistical approaches that can correct for non-ignorable non-response bias that can undermine valid inference of survey estimates leading to uncertainty in epidemic intelligence indicators. The epidemiological methods explored through this dissertation have implications on how applied epidemiologists and public health practitioners can address important limitations in surveillance infectious disease burden that can interfere with an optimal public health response.
Recommended Citation
Qasmieh, Saba, "Application of methods to population-based surveys to reduce bias in estimates of SARS-CoV-2 infection burden in the population" (2024). CUNY Academic Works.
https://academicworks.cuny.edu/sph_etds/106