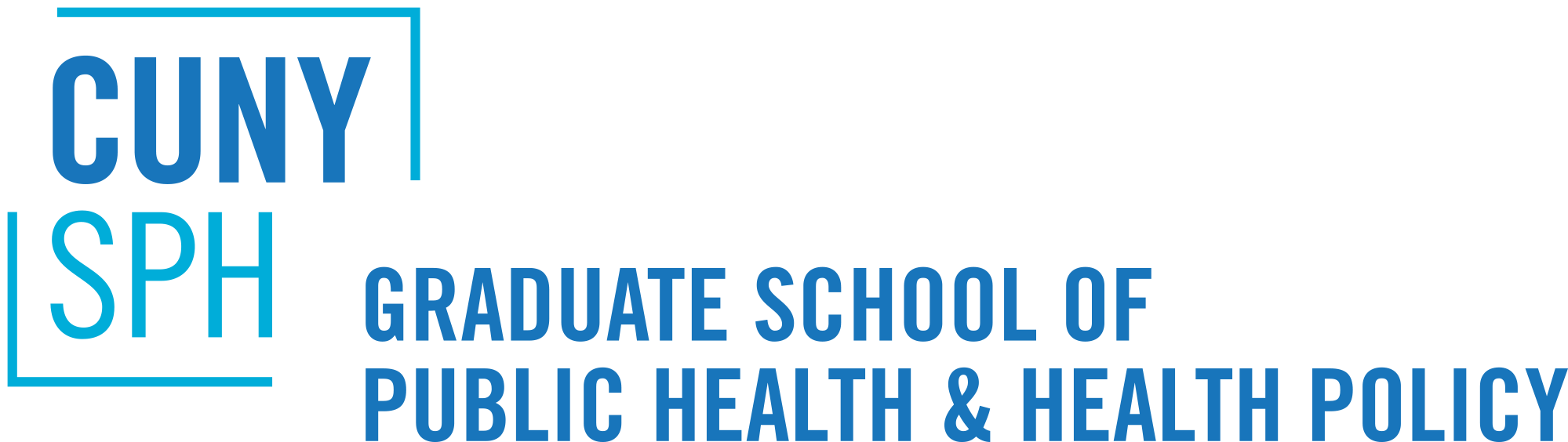
Dissertations and Theses
Date of Degree
6-3-2021
Document Type
Dissertation
Degree Name
Doctor of Public Health (DPH)
Department
Environmental, Occupational, and Geospatial Health Sciences
Advisor(s)
Jean Grassman
Committee Members
Sherry Baron
Emma Tsui
Niklas Krause
Robert Harrison
Subject Categories
Public Health
Keywords
hotel room cleaner, injury, machine learning, narrative text, occupation, task
Abstract
Abstract
Background
Hotel room cleaners are a high-risk occupation for musculoskeletal disorder (MSD) injuries among U.S. hotel workers. The Bureau of Labor Statistics (BLS) publishes occupational injury rate data for Maids and Housekeeping Cleaners, the Standard Occupation Classification closest to the job of a hotel room cleaner, and for the hotel industry, yet the BLS does not cross reference injury rate data by occupation and industry. This lack of occupational injury surveillance data limits MSD injury prevention and intervention efforts targeting this high-risk occupation in the hotel industry. Workers’ compensation (WC) data is another source of administrative data with potential for occupational injury research. Narrative text fields for occupation and injury incident descriptions may contain valuable information about job title and task at time of injury, yet present challenges to accurately classify WC claims data for coding into useable data for quantitative analysis. Beyond worker survey results, gaps exist in the peer-reviewed literature about hotel room cleaner tasks associated with MSD injuries.
Method
A quantitative retrospective record review of administrative data from the California Workers’ Compensation Information System (WCIS) of hotel room industry employee claimants with musculoskeletal disorders (MSDs) was performed. Artificial intelligence (AI) methods were applied to identify narrative text data extracted from the WCIS dataset, demonstrating the feasibility of utilizing natural language processing (NLP) techniques to transform narrative text data into coded data suitable for quantitative data analysis. This proof of concept study explores and tests list maintenance and machine learning processing methods to classify narrative text WC data for occupation and task at time of injury. Definitions from the Cal/OSHA 3345 Hotel Housekeeping Musculoskeletal Injury Prevention standard serve as a framework for the classification categories of occupation and task. Analyses were performed to describe the frequency of musculoskeletal disorders by demographic and work characteristics, and injury prevalence.
Results
A total of 12,125 claims for the occupation of hotel room cleaner were identified in California workers’ compensation data for years 2007-2016 among 23,070 hotel industry MSD injury claims. Hotel room cleaner claimants account for 52.6% of the hotel industry claims, which is 2.3 times the expected proportion given that they represent 23% of a hotel’s workforce. All claimants were female. Upper extremities (40.5%) was the leading part of body category, followed by trunk, with low back area (27.1%) and shoulders (14.3%) the leading individual parts of body injured. Task at time of injury was identified for a total of 2,628 MSD injury claims stratified by nature of injury, cause of injury and part of body variables for years 2012-2016. Injury prevalence when stratified by seven task categories varied with each task. Cleaning surfaces accounted for the highest proportion of strain claims. Pushing or pulling ranked first as cause of injury for cart handling (61.5%), moving furniture (45.9%) and vacuuming (34.7). Lifting was the most frequent cause of injury for collecting/disposing trash (48.8%), moving linen/supplies (32.1%) and bedmaking (26.5%). For the task dataset overall, the leading causes of injury were “strain or injury by” (28.1%), “pushing or pulling” (24.8%) and “lifting” (17.8%). These three causes of injury were equally distributed as the leading causes of injury for bedmaking, together accounting for 76% of this task at time of injury category.
Conclusion
The use of definitions from Cal/OSHA 3345 as a framework for classifying claims data by occupation, job title and task was pivotal for sorting and coding the data, making it accessible for the application of artificial intelligence and data management methods. This data is now available for researchers to monitor and evaluate the impact of the Cal/OSHA 3345 Hotel Housekeeping Injury Prevention standard, promulgated in 2018, specifically and in general to apply to other industries for occupational injury surveillance. In addition, task-specific findings provide insight into areas for further development of worksite intervention studies as part of injury prevention and control efforts.
Recommended Citation
Vossenas, Pamela, "The Intersection of Industry, Occupation, and Job Tasks with Hotel Room Cleaner Musculoskeletal Disorder Injuries: A Methods Approach to the Analysis of California Workers’ Compensation Data" (2021). CUNY Academic Works.
https://academicworks.cuny.edu/sph_etds/68