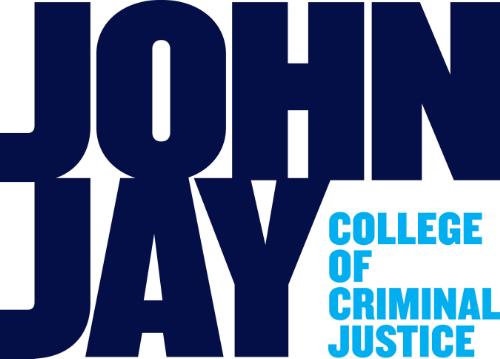
Publications and Research
Document Type
Presentation
Publication Date
4-22-2016
Abstract
Adverse interactions between police and the public harm police legitimacy and produce high costs due to harms to both officers and the public as well as litigation. Early intervention systems (EIS) that flag officers considered most likely to be involved in one of these adverse situations are an important tool for police supervision and for targeting of interventions such as counseling or training. However, the EIS that exist are often not data-driven and are based on supervior intuition. We have developed a prototype data-driven EIS that uses a diverse set of data sources from the Charlotte-Mecklenburg Police Department and machine learning techniques to more accurately predict the officers who will have an adverse incident. The predictive approach is able to significantly improve accuracy compared with their existing EIS: preliminary results indicate a 30-50% reduction in false positives and a 10-20% increase in true positives
Included in
Criminology and Criminal Justice Commons, Law Enforcement and Corrections Commons, Social Control, Law, Crime, and Deviance Commons
Comments
Presentation from 4/22 NACOLE Symposium; resources available at http://www.jjay.cuny.edu/nacole-academic-symposium